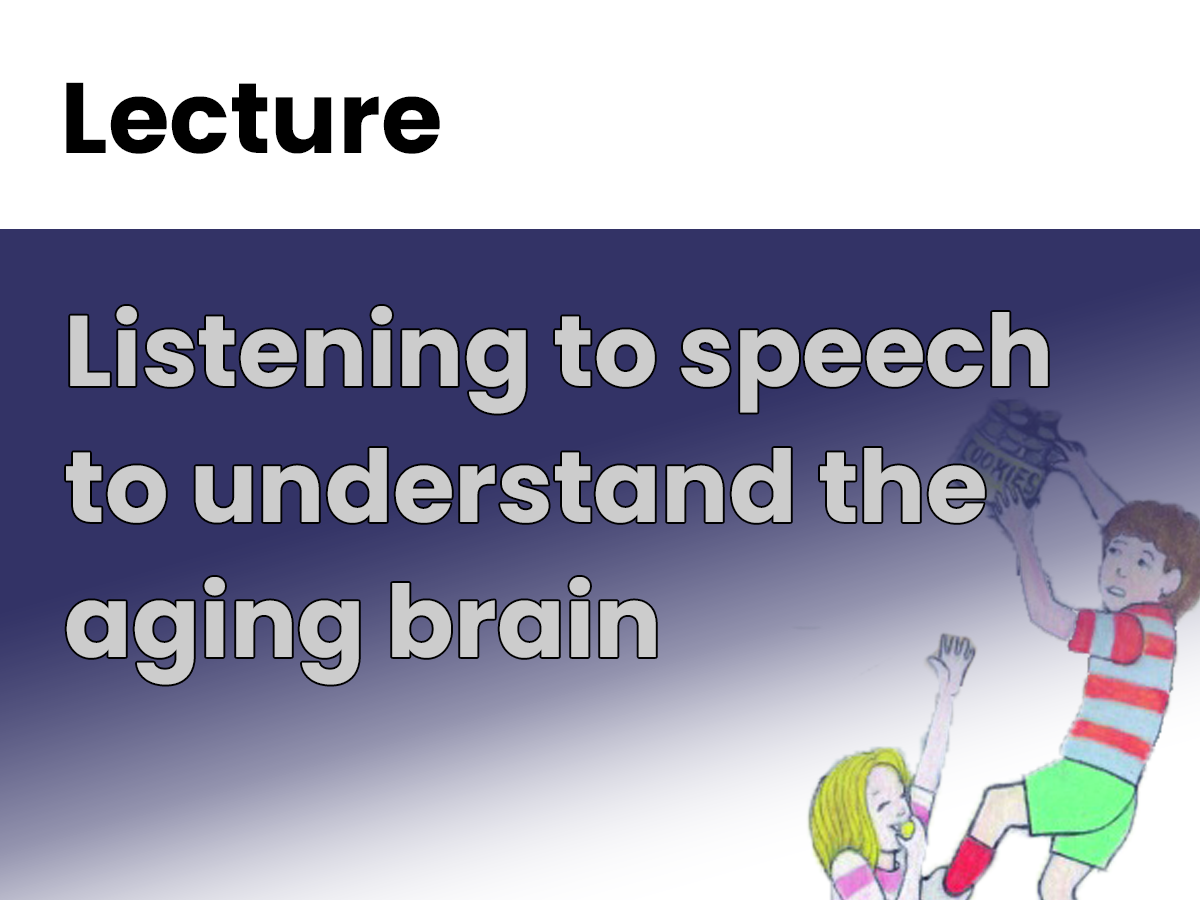
Listening to speech to understand the aging brain
Detecting Alzheimer’s disease early is the key to preventing it. Dementia is a destructive disease, meaning that it causes permanent damage to our brains that is irreversible. To reduce our risk for developing it, we can modify our lifestyles by (for example) eating healthier foods or exercising regularly, however this only reduces future risk and doesn’t prevent it entirely. The good news is that there are a number of drugs that have either been recently approved or will soon be approved that are intended to also reduce this risk, however the downside is that they only work if you have a marker of Alzheimer’s disease called “amyloid” and not if you already have dementia: this stage where a patient has high amyloid levels and is cognitively normal is called the “preclinical” stage of Alzheimer’s.
What complicates things is that even if you have high amyloid levels, you aren’t guaranteed to develop Alzheimer’s disease or even any cognitive impairment at all. Many otherwise completely healthy older adults live their whole lives in the “preclinical” stage and never go on to develop cognitive impairment. So, to test if these new drugs work, we need to measure cognitive functioning over time to see if patients are noticeably declining, and to differentiate between groups that have received the drug and those that have not. Unfortunately, all of our current methods of measuring cognitive decline perform poorly at the preclinical stage, and identify individuals in this stage as cognitively normal only until impairment is fairly obvious.
This is where my study comes in. Some previous research has shown that speech behavior, measured using simple picture description or story recall tasks, can actually differentiate between people with high amyloid and normal amyloid levels. Some of the metrics used include the amount of time it takes to recall a word or phrase, the duration of pauses or “searching” words like “um” or “uh”, and the complexity of words that are chosen. I use these metrics, plus some new ones including spectral analysis of the audio file and sentiment analysis using natural language processing, to identify patterns between a) cognitively normal participants with normal amyloid, b) cognitively normal participants with high amyloid (preclinical Alzheimer’s), and c) mildly cognitively impaired participants with high amyloid (but not full dementia). From there, we can use these findings to improve screening procedures to potentially identify preclinical Alzheimer’s disease even in a remote or virtual setting.